A second post on the book, Noise, by Kahneman, Sibony, and Sunstein. For the first see here. The authors are experts in human judgment and they have a few useful comments in defense of robots and their judgments.
Arguments Against, And For, Robots
Noise is a book about the problems of variability in human judgment. It makes sense the authors would look at the alternatives. A big one is to let robots, algorithms, make judgments instead. There are some reasonable arguments against rigid applications of robot intelligence that don’t have human input. Still, a lot of these anti-robot arguments come down to humans setting up silly rules up-front, training AI to follow these, and the robots faithfully carrying out the silly rules. I.e., it is the human intelligence that is the problem. The AI isn’t using its intelligence, but following human rules.
To counter this the authors note that:
…although a predictive algorithm in an uncertain world is unlikely to be perfect, it can be far less imperfect than noisy and often-biased human judgment.
Kahneman, Sibony, and Sunstein (2021)
The Outcomes of Algorithms Can Be Checked
The authors make the excellent point that it is a lot easier to test what algorithms are doing. For example, imagine you worry about bias, you can run a load of items through the algorithm and see what comes out. This will allow you to back out what the algorithm is ‘thinking about’ when it makes its judgments. You can’t do that with human beings. Humans won’t give you enough responses before they refuse to answer the questions. Humans will answer inconsistently (exhibit more noise) which will make your reading on bias harder to obtain. Plus, humans are likely to judge differently when they know they are being observed. Robots are true to themselves whoever is watching.
So in some ways, an algorithm can be more transparent than human beings are.
Kahneman, Sibony, and Sunstein (2021)
The key point is that you shouldn’t reject algorithms without trying to work out how bad humans are at the same task.
If algorithms make fewer mistakes than human experts do and yet we have an inuitive preference for people, then our intutive preferences should be carefully examined.
Kahneman, Sibony, and Sunstein (2021)
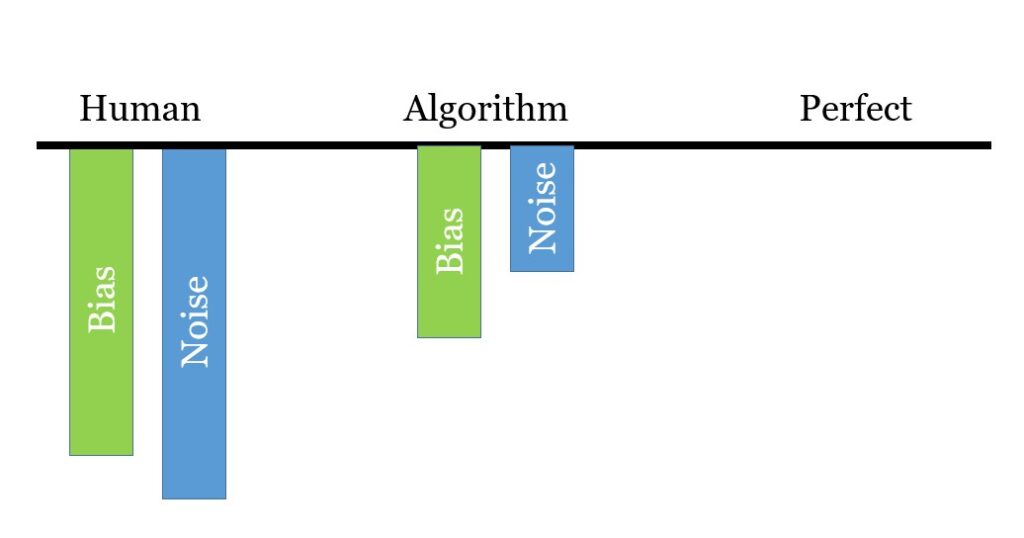
Who Hates Algorithms?
When thinking about attacks on algorithms think of who is doing the attacking. Algorithms are just rules on steroids, admittedly sometimes with a dash of je ne sais quoi. People who are in charge don’t like rules holding them back.
In general, people in positions of authority do not like to have their discretion taken away.
Kahneman, Sibony, and Sunstein (2021)
If an expert tells you that things were much better when they were just left to do whatever they wanted to do I have a plan. Think for a moment, and then say bullshit. You can cut out the thinking step if you are in a rush.
In Defense Of Robots And Their Judgments
There is nothing free in the world. Algorithms certainly have problems but what is the point of saying that algorithms have problems compared to an unachievable ideal of perfection? It is obviously true.
Yet, human judgment is noisy and biased; algorithms will very often be less noisy. (They don’t get tired etc..) Bias in robot judgments will depend upon a lot of things, for example, the training data and the algorithm itself but should we reject algorithms out of hand? No, of course we shouldn’t. In defense of robots and their judgments, I will say that they could potentially be fair and consistent. They aren’t necessarily always but their problems can be worked on. Don’t believe technophobes who in the past probably thought gramophones who subvert the youth or vaccines were just a sinister plot to keep people alive.
For related posts see here, here, here, here, and here. And for my page on public policy and decision-making see here. And for a discussion of machine learning and marketing see here.
Read: Daniel Kahneman, Olivier Sibony, and Cass R. Sunstein (2021) Noise: A Flaw in Human Judgment, Little, Brown and Company