Tim Harford’s latest book targets cynicism about data. The villain of the piece is Darrell Huff. Huff is the author of a fun book on lying with statistics, see here for more. Harford argues we are in danger of writing off the value of statistics. Instead, we need to develop clearer ideas for understanding statistics.
Smoking Stats And Cynicism About Data
Harford contrasts two different approaches to statistics. A key story he focused upon was attitudes to smoking in the mid-twentieth century. In the evil corner is Darrell Huff, who is cynical about data. Huff tries to rubbish any connection between death and smoking. (It is a fascinating story that does make one look a little differently at Huff and his ‘fun’ book). In the medicine corner were a pair of doctors who were studying if smokers died early. One of the pair responded nicely to someone who accused the doctor of trying to stop the accuser from smoking. The gist of the response was that the doctor was just looking at what happened whether or not the man continued to smoke. If the man smoked and died that was fine with the doctor as the man would provide a useful data point. (I guess anti-vaxxers are doing us a minor public service by providing a control group).
To Harford gullibility is a concern but cynicism is more so.
I worry about a world in which many people will believe anything, but I worry far more about one in which people believe nothing beyond their own preconceptions.
Harford, 2021, page 14
I take Harford’s point that we have a problem when a lot of people refuse to believe any data at all. Nothing can change their minds.
Good Advice
Harford has ten simple rules. One of these is to check how you feel about data. If you are happy to hear the result be especially careful. If someone told me that middle-aged professors born in England were the group most likely to win sexiest man alive then I, unfortunately, should be a bit suspicious. It sounds, and is, too good to be true.
Another good piece of advice is:
.. if we don’t understand the definition, then there is little point in looking at the numbers…..
.. our confusion often lies less in numbers than in words.
Harford, 2021, page 84
I see this constantly in academia. Scholars often seem to pay great attention to their model but grab any old nonsense data to throw into the model. As such, I have a piece of advice in the spirit of Harford. If you don’t understand what your dependent variable represents exactly, then there isn’t much point in looking at what might influence your dependent variable.
Theory Matters For Empirical Work
A lot of people might think they let the data speak but that doesn’t really make sense.
… a theory-free analysis of mere correlations is inevitably fragile. If you have no idea what is behind a correlation you have no idea what might that correlation to break down.
Harford, 2021,page 156
Theory has led us to many problems but so have attempts at theory-free thinking. This is partly because without explicit theory we tend to supply our own implicit theories; basically our prejudices, unjustified assumptions, and intellectual quirks. The data detectives that Harford wants to train to conduct empirical investigations need to appreciate the value of theory.
The Average Passenger Gets A Below Average Experience
One of the most interesting statistical quirks Harford points to is how we might report service levels. This has obvious customer experience implications. A train company might report how busy its trains are. This seems like a sensible thing to do but in some ways it is misleading. It is misleading because the trains themselves don’t care how busy they are. A train doesn’t have feelings. (Is there an AI scholar working on that oversight?) Instead, passengers care how busy the train they are on is. The passenger perspective gives the true measure of customer experience. You might think, what’s the difference? There is a big difference.
The average train might be empty but the average passenger might be on a crowded train. This is because passengers are all crowding onto the same trains, e.g., at rush hour. The fact that one train has a lot of passengers on it is what makes passengers feel crowded. The math is quite simple. The key thing to think about is that the train isn’t really having an experience that we care about. (Don’t worry the train will be alright). We want to measure the passenger experience. Unless we are really bad at customer experience measurement and just want a half-decent number to wave around. The train-based view might be deliberately chosen to look good but not for any other reason.
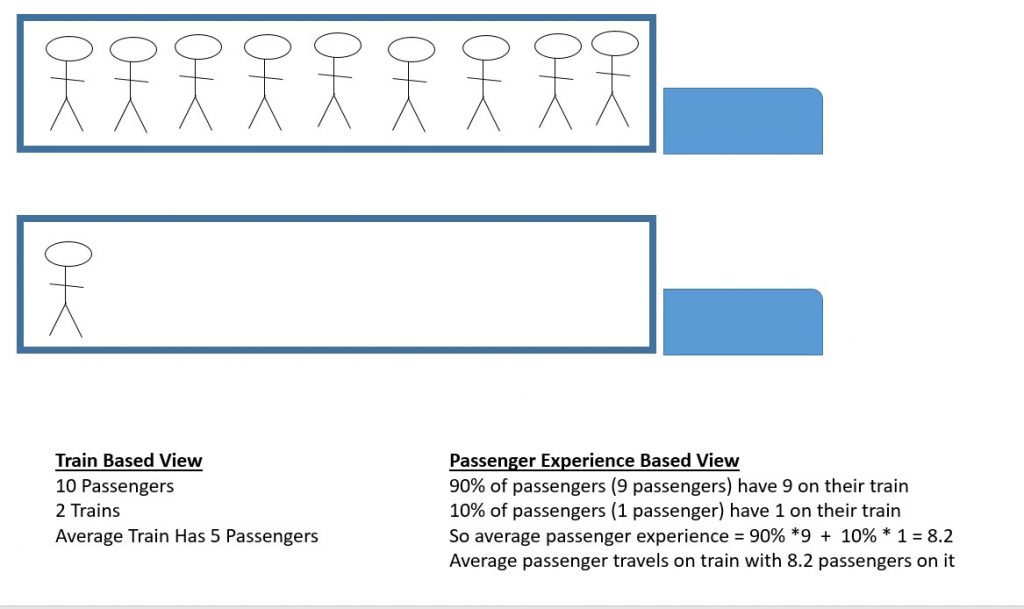
Florence Nightingale
Who knew how to use data? Florence Nightingale. (For those who don’t know she is famous in the UK for her work improving the medical conditions of soldiers in the Crimean war). Harford gives lots of excellent stories about how she marshalled data to make her case. I would say we should put her on the currency but she was already the first woman to earn her spot on the pound sterling. (Obviously, the queen is also on it but my point stands). Her story tells us how being data-savvy can help improve the world.
For more on data visualization see here.
Read: Tim Harford (2021) The Data Detective: Ten Easy Rules to Make Sense of Statistics, Penguin,